Welcome to the first video in this new playlist that is devoted to Topic 5 in the FRM. Topic 5, Market Risk, is the first topic in Part 2. We will start here by comparing normal to lognormal VaR and, specifically, we are going to generalize to absolute VaR. Absolute VaR generalizes the relative VaR so it's the complete version of VaR. The key thing that we are going to do here is look at four different use cases so we can compare normal VaR to lognormal VaR in the single-period case. Normal is when we assume that the arithmetic returns are normally distributed and lognormal is when we assume that the geometric returns are normally distributed.
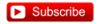
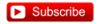