AIMs: Define default correlation for credit portfolios. Identify drawbacks in using the correlation-based credit portfolio framework. Assess the effects of correlation on a credit portfolio and its Credit VaR.
Questions:
309.1. Consider a pair of two speculative credits, rated BB and BB-, with default probabilities respectively of 2.00% and 3.00%. If their joint default probability is 0.40%, which is nearest to the implied default correlation?
a. Zero
b. 0.0830
c. 0.1424
d. 0.3750
309.2. According to Malz, each of the following is an important implication of default correlation in models of portfolio credit risk EXCEPT for:
a. Default correlation is hard to measure or estimate using historical default data
b. Default correlation exhibits too much sway on ("has a tremendous impact on") the credit portfolio's expected loss
c. Default correlations are small in magnitude such that an "optically" small correlation can have a rather large impact
d. The problem created by portfolio with (n) credits which require n*(n-1) pairwise correlations is often solved by assuming all pairwise correlations equal to a single parameter, but that parameter must be non-negative
(Source: Allan Malz, Financial Risk Management: Models, History, and Institutions (Hoboken, NJ: John Wiley & Sons, 2011))
309.3. Assume a portfolio with a total principal value of $1.0 billion divided into n = 20 positions where each position has a default probability of 1.0% and the positions are uncorrelated, as follows:
a. The initial assumption (above) imply a 95% CVaR of $40.0 million
b. An increase in granularity, from n=20 to n=100 positions, will correspond to a DECREASE in the 95% CVaR
c. As granularity increases to a very large number of independent small positions (n --> ∞), the 95% CVaR tends toward $990.0 million
d. An increase in the default correlation corresponds to an DECREASE in the 95% CVaR
Answers:
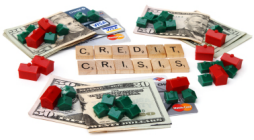
Questions:
309.1. Consider a pair of two speculative credits, rated BB and BB-, with default probabilities respectively of 2.00% and 3.00%. If their joint default probability is 0.40%, which is nearest to the implied default correlation?
a. Zero
b. 0.0830
c. 0.1424
d. 0.3750
309.2. According to Malz, each of the following is an important implication of default correlation in models of portfolio credit risk EXCEPT for:
a. Default correlation is hard to measure or estimate using historical default data
b. Default correlation exhibits too much sway on ("has a tremendous impact on") the credit portfolio's expected loss
c. Default correlations are small in magnitude such that an "optically" small correlation can have a rather large impact
d. The problem created by portfolio with (n) credits which require n*(n-1) pairwise correlations is often solved by assuming all pairwise correlations equal to a single parameter, but that parameter must be non-negative
(Source: Allan Malz, Financial Risk Management: Models, History, and Institutions (Hoboken, NJ: John Wiley & Sons, 2011))
309.3. Assume a portfolio with a total principal value of $1.0 billion divided into n = 20 positions where each position has a default probability of 1.0% and the positions are uncorrelated, as follows:
- Portfolio value = $1,000,000,000
- Number of positions (n) = 20; each position is $50.0 million
- Each position's default probability (PD) = 1.0%
- Default correlation = zero
- Credit value at risk (CVaR) confidence level = 95.0%
a. The initial assumption (above) imply a 95% CVaR of $40.0 million
b. An increase in granularity, from n=20 to n=100 positions, will correspond to a DECREASE in the 95% CVaR
c. As granularity increases to a very large number of independent small positions (n --> ∞), the 95% CVaR tends toward $990.0 million
d. An increase in the default correlation corresponds to an DECREASE in the 95% CVaR
Answers: