The three approaches are 1. Parametric; aka, analytical; 2. Historical simulation; and 3. Monte Carlo simulation (MCS). The parametric approach assumes a clean function, the other two work with messy data. Historical simulation is betrayed by a histogram, MCS is betrayed by a random number generator. In terms of the PARAMETRIC APPROACH (the 1st of three approaches), the key question is, "How do we estimate current volatility?" and we can take three basic approaches to this question.
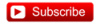
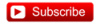
Last edited: