gargi.adhikari
Active Member
Hi,
In reference to R15.P1.T2.DIEBOLD_CH7_PARTIAL_AUTO-CORRELATION :-
I am having a bit of a confusion with the verbiage circled in Red below.
What I have managed to understand on this topic is that :- the PACF ( Partial Auto Correlation) allows us to identify the "Order" of the AutoRegression(AR) Models - AR (1 ) vs. AR ( 2) vs. AR( 3) ...etc...and in PACF we remove the effects of the in between lag variables ...
But Partial Auto Correlation itself is not Auto Regression..or is it...? OLS Regression is just used to find the value of the coefficients I guess ...??

Please pardon my ignorance on this topic




...trying to understand the best I can on this one... and as always many thanks for taking the time to share insights on this... 


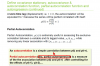
In reference to R15.P1.T2.DIEBOLD_CH7_PARTIAL_AUTO-CORRELATION :-
I am having a bit of a confusion with the verbiage circled in Red below.
What I have managed to understand on this topic is that :- the PACF ( Partial Auto Correlation) allows us to identify the "Order" of the AutoRegression(AR) Models - AR (1 ) vs. AR ( 2) vs. AR( 3) ...etc...and in PACF we remove the effects of the in between lag variables ...
But Partial Auto Correlation itself is not Auto Regression..or is it...? OLS Regression is just used to find the value of the coefficients I guess ...??


Please pardon my ignorance on this topic









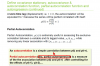
Last edited: